指数族
指数族可以描述许多常见分布(正态分布,二项分布,泊松分布等),且其与 sufficiency 以及最优 data reduction 密切相关
The model forms an s-demensional exponential family if each has density of the form:
- are called the natural parameters
- are its sufficient statistics
- is the log-partition function (logarithm of a normalization factor)
是一个与参数无关的基准测度,can be “absorbed” into the measure
【例】指数分布
对应概率密度:
一维指数族:
中对应的符号可以给两者任意一个。因此指数族的参数化不是唯一的
【例】Beta 分布
对应概率密度:
- for
可以看出一般情况下,几个统计量 解决几个“未知参数”
在指数族的定义中,很自然地可以把 当成新的参数 来确定密度函数,这样密度函数的主体就是未知参数 的线性组合,由此引入指数族的自然形式(也称规范形式):
canonical exponential family
An exponential family is in canonical form when the density has the form
The natural parameters is instead of .
【例】Gaussian 分布
概率密度:
将可以产生有效的概率密度的 集合定义为自然参数空间:
For each , there exists a normalizing constant such that . Equivalently,
任意自然形式指数族可写作 且
指数族的秩
is identifiable if
有两种情况可以 reducing the dimension of an s-dimensional exponential family
- , 满足仿射等式约束
这里 线性相关。 可实现降维
由于 for any ,因此非 identifiable
- 对所有 , 满足仿射等式约束
A canonical exponential family is minimal if
- (no affine equality constraints)
- (no affine equality constraints)
设 是 s-dimensional minimal exponential family, 线性无关,则 称作 full-rank exponential family,秩为
否则称 为 curved exponential family
因此可以将指数族分为 3 类:
- Non-minimal (有线性关系,可降维)
【例】正态分布 , when

- Minimal & Curved (曲线指数族)
【例】正态分布 ;
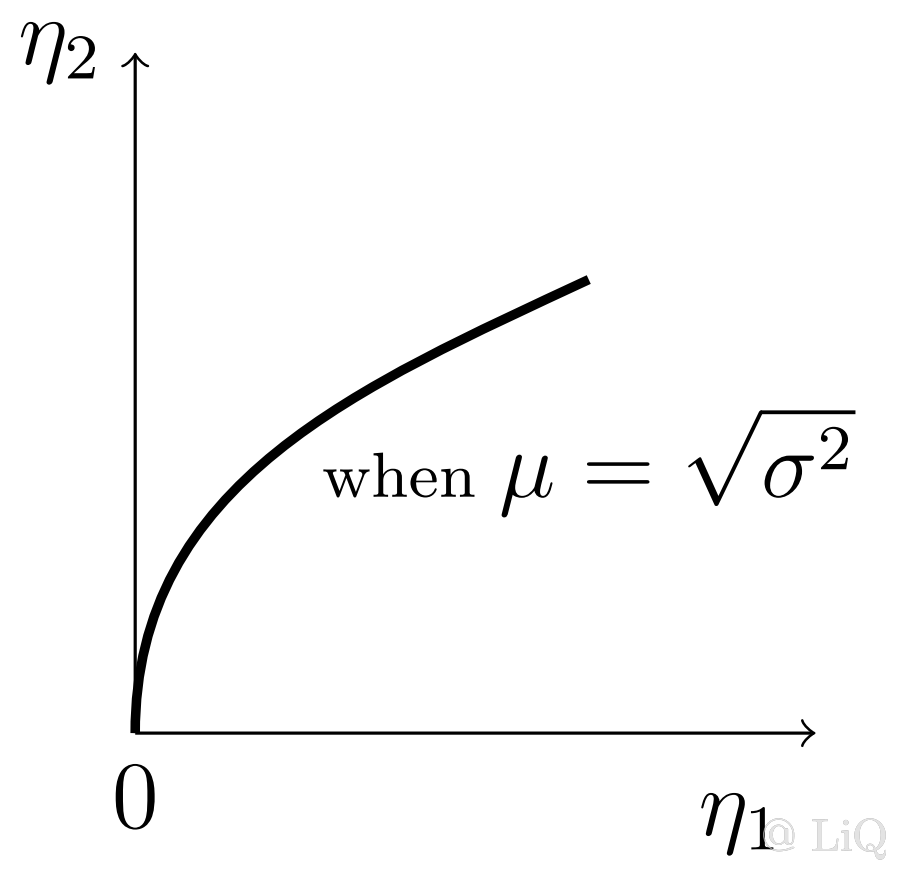
- Minimal & Full-Rank (自然参数空间 )

指数族性质
- 如果 ,则
指数相乘易证; 是充分统计量
在 上解析(无穷次可微),且积分微分运算可调换
证明利用 Laplace 变换解析性质
可通过取 的偏导数来计算充分统计量的平均值
取 ,则
同理可求任意阶矩如
参考
© 2024 LiQ
:) 由 Obsidian&Github 强力驱动